Artificial Intelligence Shaping Transport
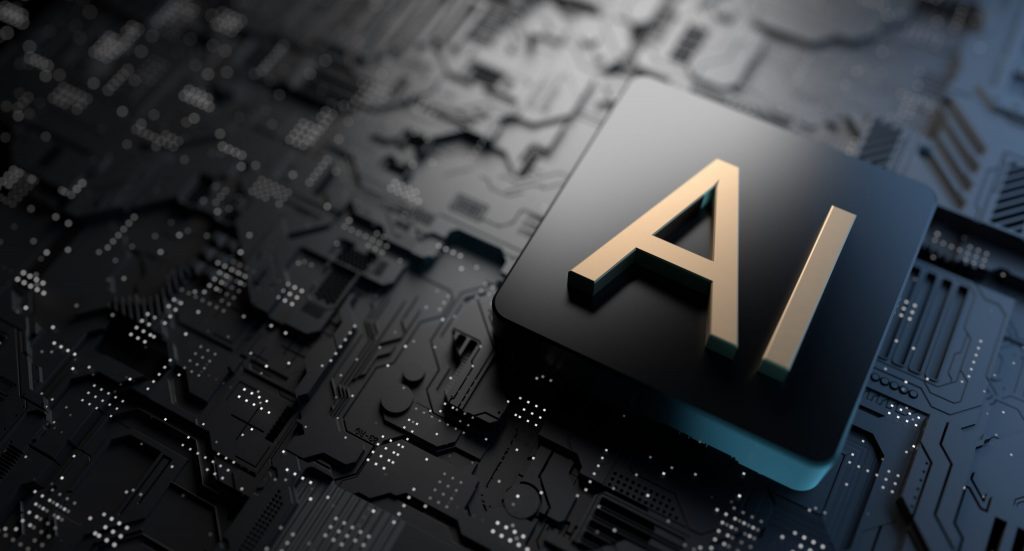
The Evolution of Machines
Life without machines today would be unimaginable, given the rapid transformation brought about by technological advances in automation, computing, and communication. During the industrial revolution, motorized machines like tractors and cranes targeted manual labor tasks, but humans still directed them. The evolution led to programmable machines, allowing us to define instructions for execution. However, challenges arise when machines face unprogrammed situations or complex instructions, like identifying a human face. Humans rely on identifiable patterns for decision-making in ambiguous situations. Translating this human intelligence into machines poses a challenge. Artificial Intelligence (AI) has been a long-standing subject of research, applied to fields like biometrics, robotics, finance, medical science, and industrial automation. Recent advances in mobile technology have introduced smart devices tracking micro-level details in our daily lives—such as fitness, food, and shopping habits. Simultaneously, they provide macro-level insights into the broader ecosystem, covering traffic conditions, weather, and the economy. This integration of AI into daily life has created a dynamic environment, shaping personal behaviors and societal systems. As technology advances, machines and AI are becoming integral to how we navigate and interact with the world.
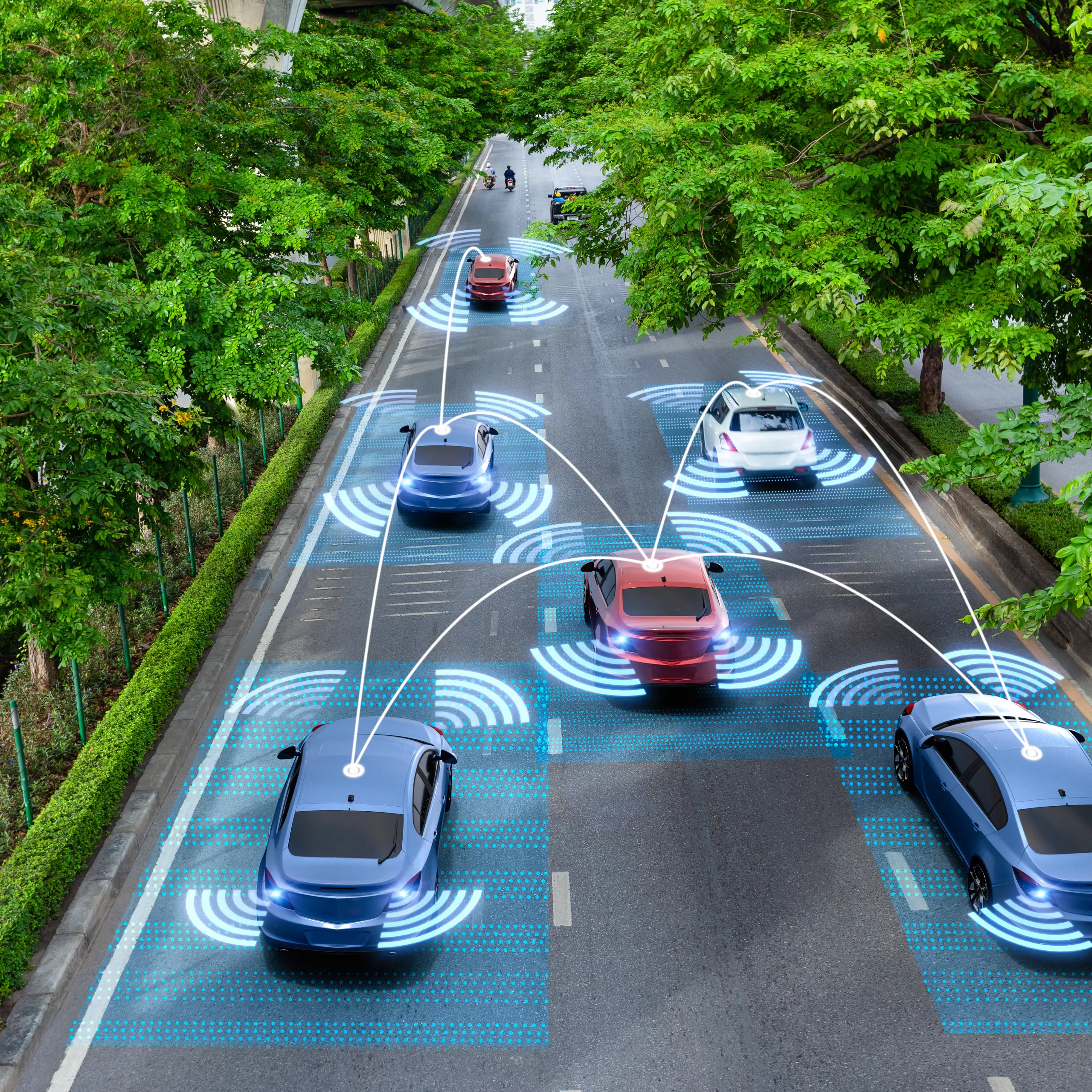
Artificial Intelligence and Machine Learnings
Artificial Intelligence (AI) is the field focused on creating machines capable of emulating human-like decision-making. Within the realm of AI, Machine Learning is a specific technique employed to train machines by enabling them to mimic human decision-making processes.
While Machine Learning is a crucial process for imparting intelligence to machines, it is important to note that it represents just one approach among various methods available in the broader landscape of artificial intelligence. Neural Networks fall under the category of Machine Learning algorithms, designed to replicate the complexity of the biological world where simple units form intricate systems of intelligence. Deep Learning, a subset of Neural Networks, involves constructing networks with multiple layers of units between input and output. This innovation is particularly noteworthy because, traditionally, machine learning required meticulous preprocessing of input data for easy consumption, a practice known as feature engineering. Teams of data scientists spent extensive hours reviewing, interpreting, and transforming large datasets. Deep Learning, by employing multiple layers of neural units, streamlines the data cleanup process within the neural network layers themselves. This shift makes it more practical to apply machine learning models to real-world problems.
To recap, Artificial Intelligence (AI) empowers machines to make decisions akin to human decision-making. Machine Learning (ML) serves as the technique for instilling intelligence in machines. The approach taken by ML mirrors how human children learn about the world. Just as parents guide a child’s learning by pointing to objects and labeling them, ML uses examples to train machines. This method allows the machines to unravel patterns autonomously. In contrast to the challenge of defining complex concepts like cars explicitly, this example illustrates how providing examples can effectively train a child’s brain. Similarly, teaching machines about cars through explicit feature descriptions would be impractical and might lead to confusion in distinguishing between various vehicle types.
As technology continues to advance, these concepts collectively propel us toward a future where machines not only mimic human intelligence but also contribute to solving complex problems and enhancing our daily lives. The ongoing synergy between human ingenuity and machine learning algorithms promises a transformative era in which AI becomes an indispensable tool for innovation and progress across diverse fields.
Source: Moveinsync